How artificial intelligence during the pandemic modified the role of a biomarker as d-dimer
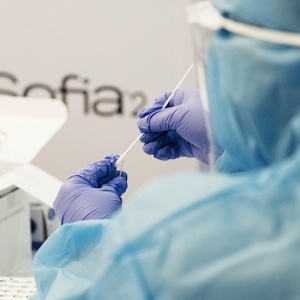
HTML: 25
All claims expressed in this article are solely those of the authors and do not necessarily represent those of their affiliated organizations, or those of the publisher, the editors and the reviewers. Any product that may be evaluated in this article or claim that may be made by its manufacturer is not guaranteed or endorsed by the publisher.
Authors
Artificial intelligence (AI) was introduced in medicine to make some difficult decision-making regarding diagnostics and/or treatments easy. Its application derives from the improvement of information obtained with computer sciences and informatics, in particular with information derived by algorithms obtained with special informatics support as machine learning. The scenario of hospital changes induced by the COVID-19 pandemic makes easy the application of AI for some clinical updates. Being lung failure with pulmonary embolism is the most common cause of death for inpatients with COVID-19, some biomarkers such as the d-dimer are constantly used associated with other clinical features in order to improve medical assistance. For this reason, d-dimer during the pandemic changed its traditional use for predictive negative value in patients with suspected pulmonary embolism and took relevance for its values giving the chance to change the intensity of anticoagulation for several inpatients. In most cases, according to data reported from several cohorts, these changes improved the morbidity and mortality of a significant percentage of inpatients with COVID-19. The International medical prevention registry on venous thromboembolism and d-dimer and modified sepsis-induced coagulopathy scores were the most used scores derived from AI and dedicated to these clinical aspects in inpatients with COVID-19. Therefore, this review was dedicated to flexible changes that we can use after d-dimer values in different clinical scenarios that vary from disseminated intravascular coagulation to pulmonary embolism to COVID-19.
How to Cite

This work is licensed under a Creative Commons Attribution-NonCommercial 4.0 International License.
PAGEPress has chosen to apply the Creative Commons Attribution NonCommercial 4.0 International License (CC BY-NC 4.0) to all manuscripts to be published.